Many people with early-stage or first-episode bipolar disorder have cognitive deficits, such as issues with visual processing and spatial memory, but those deficits are often so subtle that the disorder can go undiagnosed for years. That could change thanks to researchers at the University of Alberta who have created a machine learning model that helps identify these subtle deficits with the goal of intervening earlier.
The study was led by Jeffrey Sawalha, a doctoral student who collaborates with the U of A’s Computational Psychiatry Research Group.
Earlier diagnosis is crucial for patients with bipolar disorder as this allows psychiatrists to treat them sooner, before symptoms worsen. Evidence suggests that patients respond more strongly in the early stages to treatment with lithium.
“If you can use the cognitive test and machine learning to detect the subtle form, to prevent the progression or the emergence of a manic episode, that’s the key. We obviously cannot prevent all cases, but it may be a huge benefit for some individuals,” said Bo Cao, an assistant professor in the Department of Psychiatry and member of the computational psychiatry group, which also includes Russ Greiner and Andrew Greenshaw. All three are also members of the Neuroscience and Mental Health Institute.
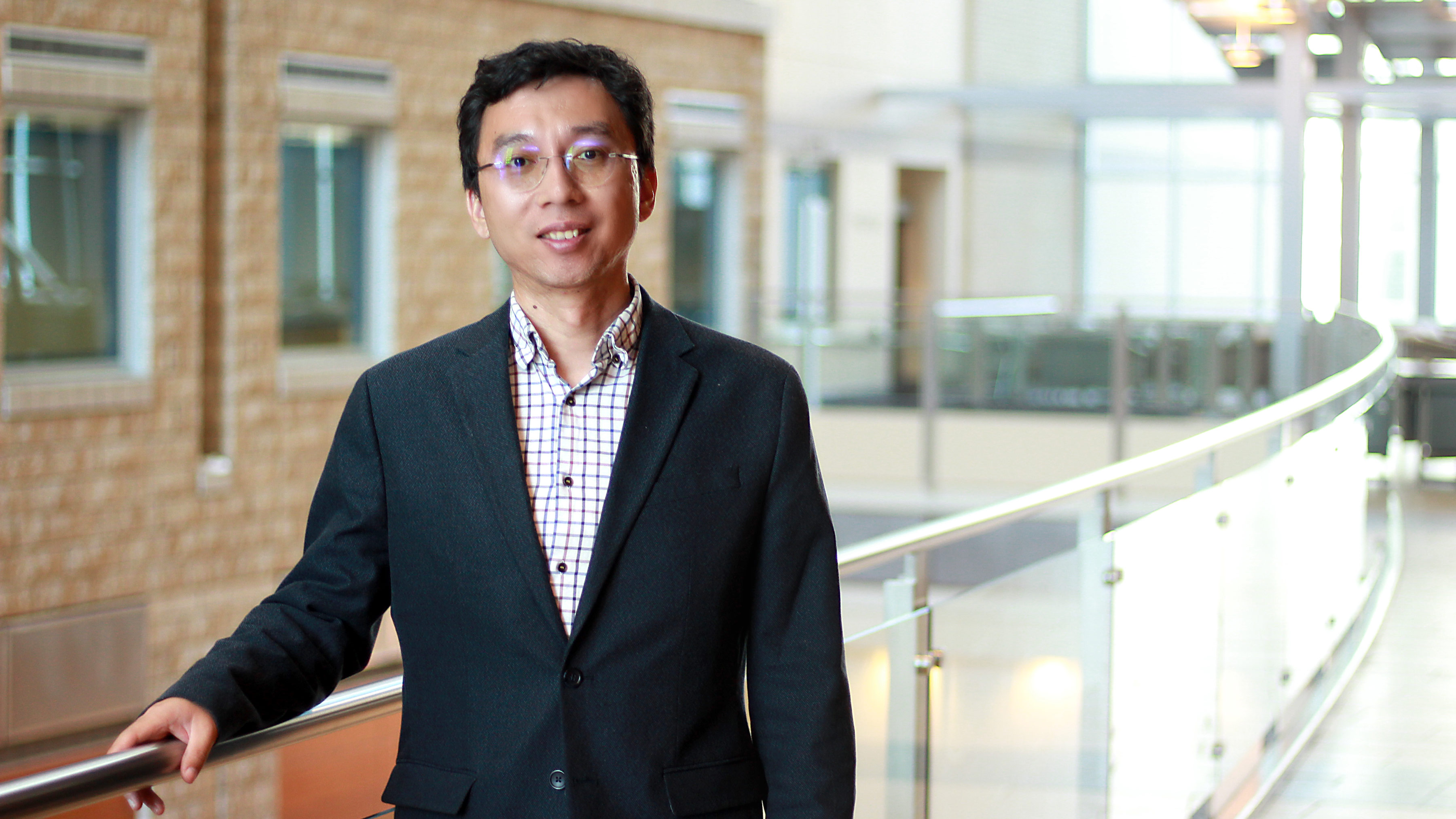
The group trained its machine learning model by comparing patients with chronic bipolar disorder to healthy control individuals, and then demonstrated that this learned model could distinguish first-episode bipolar disorder patients from healthy controls with 76 per cent accuracy. The resulting tool can examine early markers of cognitive deficits, which can then be used for early detection of bipolar disorder.
An easier, more accessible way to collect data
The U of A researchers worked with collaborators in China, who collected the data used in the machine learning model. The data were obtained using tests that targeted cognitive function. In this study the patients were supervised as they completed the tests, but most of the tests could be done virtually using a tablet.
This is in stark contrast to the current practice of obtaining information through machines such as MRIs, which provide images of the brain’s structure. According to the researchers, a simple cognitive test analyzed through machine learning can yield equally valuable data.
“If we can get the same information for pennies versus hundreds of dollars, immediately versus three weeks from now, in a stress-free environment versus a stressful hospital environment, it’s a win all around,” said Greiner, a professor in the Faculty of Science and fellow-in-residence at the Alberta Machine Intelligence Institute (Amii).
The new tool is also beneficial in monitoring a patient’s progression over time. “When it comes to followups, that information is also easier to collect. It’s an easy way of monitoring symptoms,” said Sawalha.
Fuelling machine learning models
Greenshaw stressed the importance of consistent, standardized data collection to help cultivate these types of machine learning models.
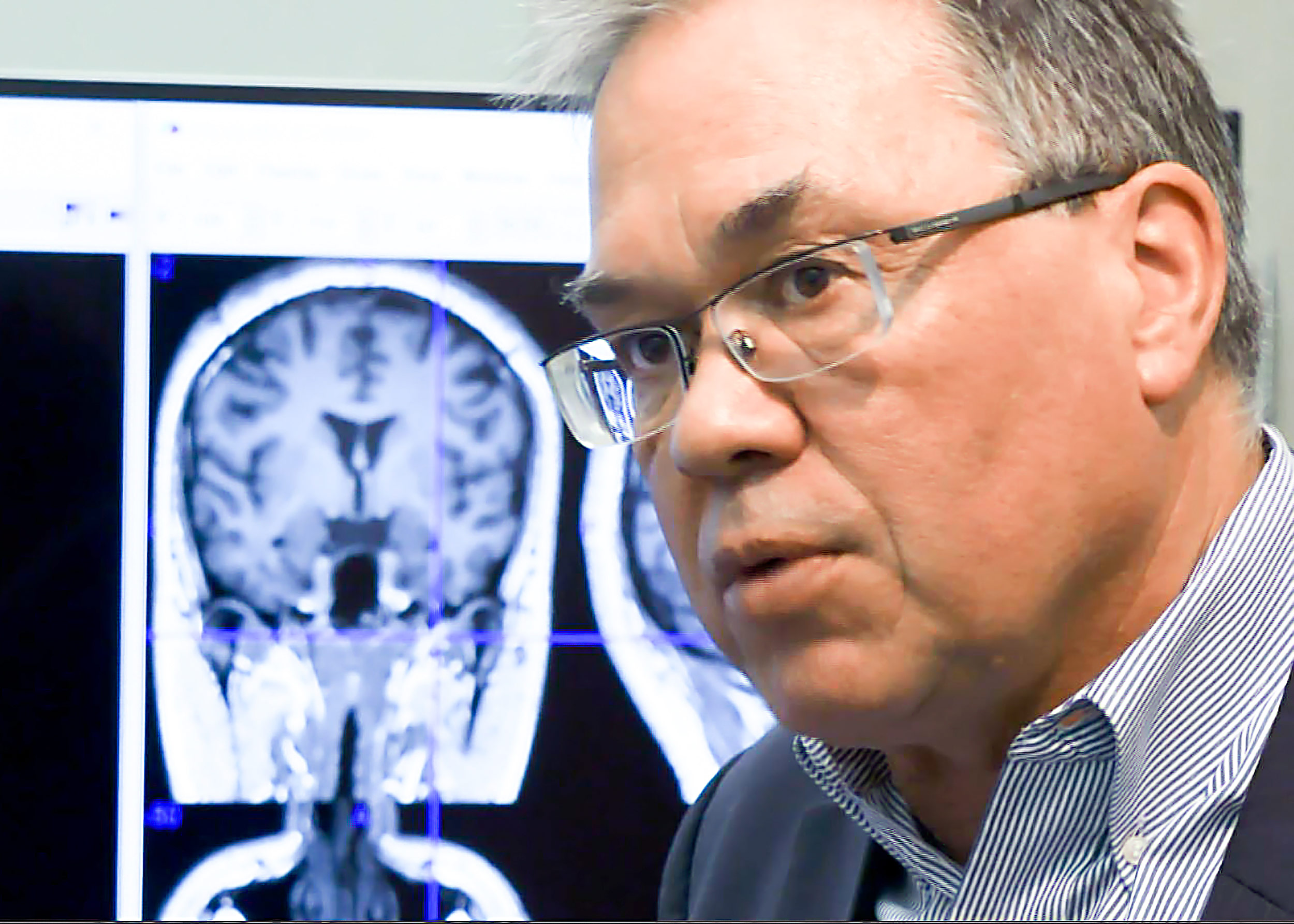
“These models are wonderful, but you need the data to build the models, and one of the things that we predict will happen with the application of machine learning is it will push the health system towards collecting better evidence-based measures,” said Greenshaw, a professor and associate chair in the Department of Psychiatry.
“For example, in other work we’ve done with antidepressant drugs, a psychiatrist trying to decide which drug to use has about a 50 per cent chance of getting it right. Applying machine learning, you can get that probability much higher, but you have to get that buy-in from physicians.”
Refining and expanding the model
The next steps in this line of research are to validate the model with a larger group of people to obtain a more comprehensive data set. The researchers are also interested in potentially using the model to look at psychotic features in schizophrenia and examining the differences between cognitive deficits in schizophrenia and bipolar disorder.
For patients with psychiatric disorders, problems with cognitive and social functioning often are as bothersome as the symptoms themselves, said the researchers. By the time conventional testing reveals the problems, the patient’s quality of life may already have deteriorated. By finding subtle cognitive deficits earlier, the new tool offers hope for a better quality of life for patients.
The study, “Individualized identification of first-episode bipolar disorder using machine learning and cognitive tests,” was published in the Journal of Affective Disorders.