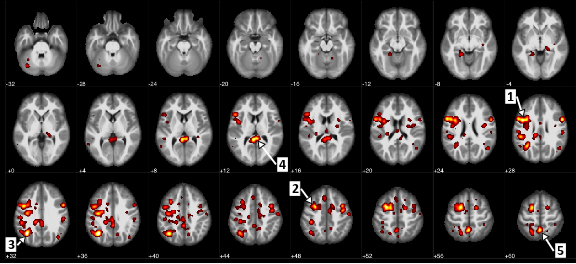
Regions of the brain that showed a statistically significant difference between patients with schizophrenia and patients without it.
A team of UAlberta researchers and IBM scientists has trained a system with machine learning to be capable of identifying schizophrenia and assessing the severity of a patient's symptoms. Using predictive computer analysis, the team has developed new algorithms to enhance psychiatrists' understanding of the disease, which could lead to tools that may objectively diagnose and assess symptoms and treatment options.
"Machine learning is a powerful tool that can help us understand complex systems like the human brain," said Mina Gheiratmand, researcher with the Alberta Machine Intelligence Institute at the University of Alberta. "Computers, unlike humans, can quickly work through vast amounts of data and consider a multitude of factors at once. With machine learning, human decision makers can gain access to this deeper level of information and analysis."
Gheiratmand is working with artificial intelligence researcher and Faculty of Science professor Russ Greiner, well known for his work in health informatics, applying statistical machine learning to health data. Greiner works to produce tools for clinicians that will assist with objective, data-driven diagnosis and treatment planning, particularly needed in areas of mental health. He has used similar techniques as those in this latest study to predict instances of ADHD in a given population.
Computational psychiatry
For their analysis, researchers used functional Magnetic Resonance Imaging (fMRI), which measures brain activity through blood flow changes in or between particular areas of the brain. Examining de-identified scans from 95 participants, researchers used machine learning techniques to develop a model of schizophrenia that identifies the connections in the brain most associated with the illness.
With author, right:"Machine learning is a powerful tool that can help us understand complex systems like the human brain." -Mina Gheiratmand
These evidence-based models are used to predict the likelihood of a previously-unseen patient having schizophrenia and to objectively assess the severity of common symptoms, including inattentiveness, bizarre thoughts or behaviour and lack of motivation.
The results of the UAlberta and IBM research demonstrated that even on more challenging neuroimaging data collected from multiple sites (different machines, across different groups of subjects etc.), the machine learning algorithm was able to discriminate between patients with schizophrenia and the control group with 74 per cent accuracy using the correlations in activity across different areas of the brain.
Clinical implications
In this ongoing collaboration, the researchers are focusing on developing models that can be interpreted by mental health professionals. The focus on explainable results not only provides insight into the areas of the brain most affected by schizophrenia but also enables psychiatrists to be confident of the factors that informed the computer's assessment.
"The ultimate goal of this research effort is to identify and develop objective, data-driven measures for characterizing mental states, and apply them to psychiatric and neurological disorders" said Ajay Royyuru, vice president of healthcare and life sciences, IBM Research. "We also hope to offer new insights into how AI and machine learning can be used to analyze psychiatric and neurological disorders to aid psychiatrists in their assessment and treatment of patients."
"This unique, innovative multidisciplinary approach opens new insights and advances our understanding of the neurobiology of schizophrenia, which will help to improve the treatment and management of the disease," said Serdar Dursun, a professor in the Department of Psychiatry. "We've discovered a number of significant abnormal connections in the brain that can be explored in future studies, and AI-created models bring us one step closer to finding objective neuroimaging-based patterns that are diagnostic and prognostic markers of schizophrenia."
In future studies, researchers will continue to investigate areas and connections in the brain with significant links to schizophrenia. They will also further improve their learned models by conducting machine learning on larger datasets and by exploring ways to extend these techniques to other psychiatric disorders such as depression and post-traumatic stress disorder.