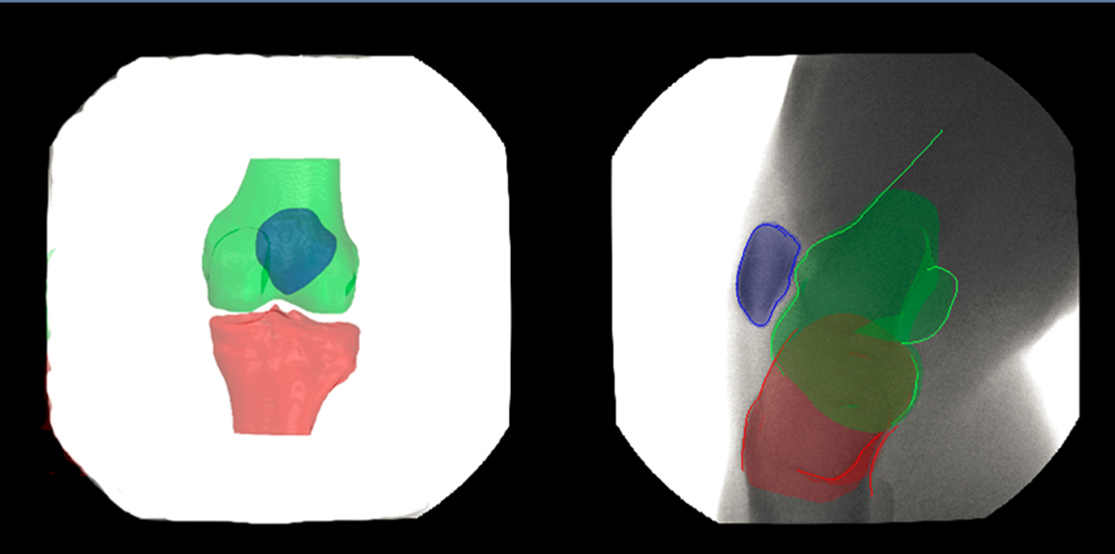
New technology by UAlberta computing scientists is helping to look at joints in motion. Photo Credit: Pierre Boulanger
New technology from UAlberta computing scientists that allow clinicians to visualize actual movement inside the human body has great potential as a diagnostic tool and could become a powerful new analytical tool for understanding body dynamics.
The system uses an automated algorithm that can register a patient specific bone model extracted from a CT imaging system to videos produced by a real-time low radiation X-ray imaging system allowing clinicians to see the unique, dynamics movements of individual bones motion inside a patient's knee.
"What we are trying to do is to create a complete dynamic model of the entire knee joint in motion, including bone, muscle, and cartilage," explained Pierre Boulanger, professor at the Department of Computing Science and Cisco Chair in Healthcare Solutions. "We are now trying to use advanced neural network techniques to segment these knee structures using standard MRI and then track them using a novel real-time open MRI machine to capture the motion."
The segmentation technique uses a deep learning algorithm to segment the knee anatomy automatically from examples provided by clinicians. The new technique is based on MRI imaging, reducing the potential harmful effect of ionizing radiation produced by X-rays.
And while this study used the knee joint as a case study, this technology can be applied to any joint or any motion in the body-from your jaw to your elbow.
Physiotherapists and orthopedic surgeons can also use this technology to understand how their work affects the body and how change is made over time. This is especially useful when a patient has a problem that is dynamic, which is very hard to diagnose. "This is the beginning of a new generation of imaging tools that can create personalized models of individual patient's joints" added Boulanger.
"Ultimately, our goal is to develop patient-specific modeling, technology, and tools for use in a clinical setting," explained Boulanger, who worked with Constance Lebrun and Fateme Esfandiarpour, University of Alberta family physicians from the Glen Sather Sports Medicine Clinic on the study.
The paper, "A Structured Deep-Learning Based Approach for the Automated Segmentation of Human Leg Muscle from 3D MRI," was presented at the 2017 Conference on Computer and Robot Vision (doi: 10.1109/CRV.2017.32).