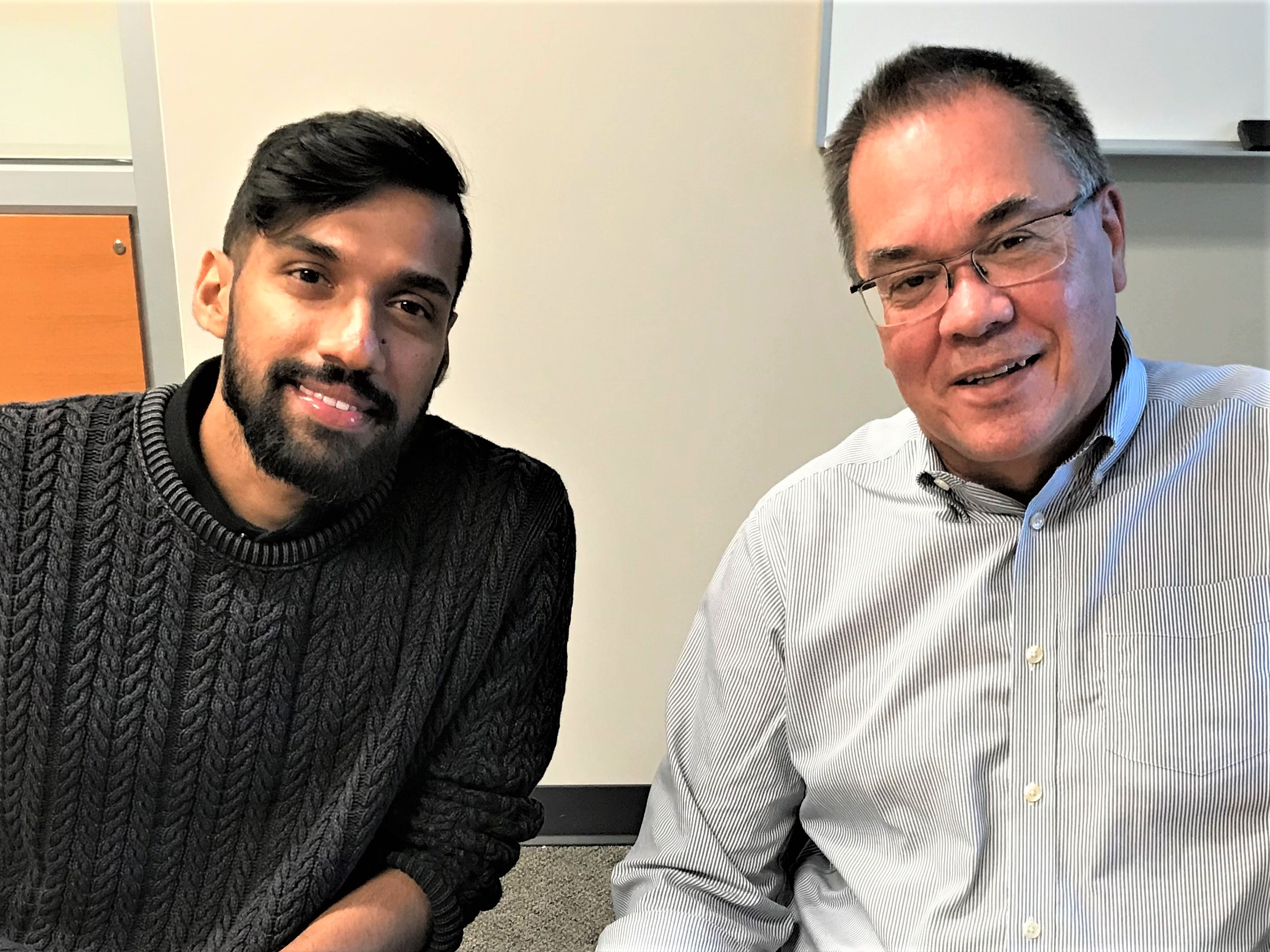
A University of Alberta researcher who harnessed the power of machine learning to develop a groundbreaking tool for identifying Schizophrenia from patients' brain scans was the subject of a feature profile on German television. Click here to view video.
Nano, a half-hour-long science program carried by Germany's 3Sat Network, recently devoted a seven-minute segment to the breakthrough research study, which was led by computer scientist Dr. Sunil Kalmady, a Postdoctoral Fellow in the U of A's Machine Intelligence Institute (Amii).
The show's producers spent several days in and around the University of Alberta campus preparing the segment, which aired this fall. Directed by Uri Schneider and hosted by Alexandra Krober, the Nano episode employed graphics and music to complement interview footage with Dr. Kalmady and three U of A Faculty members who supervised the study.
The latter included Dr. Russ Greiner, a Professor in the Department of Computing Science; Dr. Andrew Greenshaw, Professor and Associate Chair (Research) in the Department of Psychiatry; and Dr. Serdar Dursun, a Professor in the Department of Psychiatry.
"The shots they used from around the U of A campus, travelling over the North Saskatchewan River on the LRT and at DaDeO, a popular New Orleans-style diner and bar on Whyte Avenue really looked great. I thought it really helped to make the team's research on this very serious mental health issue more accessible and visually engaging," says Dr. Greenshaw.
The study - titled Towards artificial intelligence in mental health by improving Schizophrenia prediction with multiple brain parcellation ensemble-learning - was done in concert with a team of researchers from India's National Institute of Mental Health and Neuro Sciences (NIMHANS). The results were published last January in NPJ (Nature Partner Journal) Schizophrenia.
The research focused on the development of a new diagnostic tool for Schizophrenia known as EMPaSchiz - or Ensemble algorithm with Multiple Parcellations for Schizophrenia prediction - which is based on an analysis of patients' brain scans. By using EMPaSchiz, Dr. Kalmady and his research team were able to identify evidence of the disease in brain scans with a remarkable 87 per cent accuracy.
"Up to now, Psychiatrists have mainly depended on observed symptoms of Schizophrenia in the patient's behaviour, currently or in the past, along with things like their family history, to determine whether they meet the diagnostic criteria. But that's not a biologically-based assessment methodology and it can't be validated through something like a simple blood test," he says.
"As a result, there is a fair amount of subjectivity involved in these kinds of diagnostic judgements. What we did in this study is we used a modality of brain imaging called Functional MRI (Magnetic Resonance Imaging), which basically gave us a three-dimensional movie of blood oxygen level patterns in the brain. We then used this data modality to predict whether a person had Schizophrenia, and we had a very good success rate with that, of about 87 per cent."
The roughly 100 patients enrolled in the study were recruited at the NIMHANS in Bangalore - India's largest mental health hospital - over a multi-year period starting eight years ago, when Dr. Kalmady began working on his PhD research project.
"They were patients who had never been treated previously with any Antipsychotic drugs, so the brain signals we observed in their MRI scans were unaltered, which was important," he says.
"There has been at least a couple decades of MRI research in Psychiatry, so we understood a lot about what changes occur in the brain and what structures might be important, and we made use of them with this particular algorithm," he adds.
"We brought them all together in a joint fashion, and we call that approach ensemble learning. That gives us a big boost compared to just picking one of these factors. Putting them together and learning about all of them jointly greatly improves predictability, and that's what we showed in this study."
Using the machine learning tool to identify Schizophrenia early, before a patient's brain undergoes what are often lasting structural changes, offers huge potential benefits, says Dr. Greenshaw.
"Sometimes the symptoms of Schizophrenia are not that obvious, and the earlier you treat someone, the better their chances of recovery. So the first episode is really important. If you can intervene at a point when a patient is just becoming symptomatic, then you have a much better chance of catching hold of the disease, supporting patients and offering a better prognosis," he says.
"If the disease goes undetected and untreated for a while, the patient's brain changes so it's quite hard for them and there is no easy route back. But if you detect the disease before they are symptomatic, and if you could detect the risk factors, that's a huge advantage."
Since Schizophrenia is often characterized by a complex constellation of symptoms that might or might not occur simultaneously in a patient, two patients with the same diagnosis might still present with very different symptoms, increasing the odds of misdiagnosis.
"Machine learning, in this case, is able to drive an evidence-based approach that looks at thousands of features in a brain scan to lead to an optimal prediction," says Dr. Kalmady.
"Machine learning and future AI (Artificial Intelligence) are approaches that enable a multi-dimensional, data-driven inroad that captures the level of complexity and objectivity that we need to unravel the wicked problems of understanding mental illness."
Nano, a half-hour-long science program carried by Germany's 3Sat Network, recently devoted a seven-minute segment to the breakthrough research study, which was led by computer scientist Dr. Sunil Kalmady, a Postdoctoral Fellow in the U of A's Machine Intelligence Institute (Amii).
The show's producers spent several days in and around the University of Alberta campus preparing the segment, which aired this fall. Directed by Uri Schneider and hosted by Alexandra Krober, the Nano episode employed graphics and music to complement interview footage with Dr. Kalmady and three U of A Faculty members who supervised the study.
The latter included Dr. Russ Greiner, a Professor in the Department of Computing Science; Dr. Andrew Greenshaw, Professor and Associate Chair (Research) in the Department of Psychiatry; and Dr. Serdar Dursun, a Professor in the Department of Psychiatry.
"The shots they used from around the U of A campus, travelling over the North Saskatchewan River on the LRT and at DaDeO, a popular New Orleans-style diner and bar on Whyte Avenue really looked great. I thought it really helped to make the team's research on this very serious mental health issue more accessible and visually engaging," says Dr. Greenshaw.
The study - titled Towards artificial intelligence in mental health by improving Schizophrenia prediction with multiple brain parcellation ensemble-learning - was done in concert with a team of researchers from India's National Institute of Mental Health and Neuro Sciences (NIMHANS). The results were published last January in NPJ (Nature Partner Journal) Schizophrenia.
The research focused on the development of a new diagnostic tool for Schizophrenia known as EMPaSchiz - or Ensemble algorithm with Multiple Parcellations for Schizophrenia prediction - which is based on an analysis of patients' brain scans. By using EMPaSchiz, Dr. Kalmady and his research team were able to identify evidence of the disease in brain scans with a remarkable 87 per cent accuracy.
"Up to now, Psychiatrists have mainly depended on observed symptoms of Schizophrenia in the patient's behaviour, currently or in the past, along with things like their family history, to determine whether they meet the diagnostic criteria. But that's not a biologically-based assessment methodology and it can't be validated through something like a simple blood test," he says.
"As a result, there is a fair amount of subjectivity involved in these kinds of diagnostic judgements. What we did in this study is we used a modality of brain imaging called Functional MRI (Magnetic Resonance Imaging), which basically gave us a three-dimensional movie of blood oxygen level patterns in the brain. We then used this data modality to predict whether a person had Schizophrenia, and we had a very good success rate with that, of about 87 per cent."
The roughly 100 patients enrolled in the study were recruited at the NIMHANS in Bangalore - India's largest mental health hospital - over a multi-year period starting eight years ago, when Dr. Kalmady began working on his PhD research project.
"They were patients who had never been treated previously with any Antipsychotic drugs, so the brain signals we observed in their MRI scans were unaltered, which was important," he says.
"There has been at least a couple decades of MRI research in Psychiatry, so we understood a lot about what changes occur in the brain and what structures might be important, and we made use of them with this particular algorithm," he adds.
"We brought them all together in a joint fashion, and we call that approach ensemble learning. That gives us a big boost compared to just picking one of these factors. Putting them together and learning about all of them jointly greatly improves predictability, and that's what we showed in this study."
Using the machine learning tool to identify Schizophrenia early, before a patient's brain undergoes what are often lasting structural changes, offers huge potential benefits, says Dr. Greenshaw.
"Sometimes the symptoms of Schizophrenia are not that obvious, and the earlier you treat someone, the better their chances of recovery. So the first episode is really important. If you can intervene at a point when a patient is just becoming symptomatic, then you have a much better chance of catching hold of the disease, supporting patients and offering a better prognosis," he says.
"If the disease goes undetected and untreated for a while, the patient's brain changes so it's quite hard for them and there is no easy route back. But if you detect the disease before they are symptomatic, and if you could detect the risk factors, that's a huge advantage."
Since Schizophrenia is often characterized by a complex constellation of symptoms that might or might not occur simultaneously in a patient, two patients with the same diagnosis might still present with very different symptoms, increasing the odds of misdiagnosis.
"Machine learning, in this case, is able to drive an evidence-based approach that looks at thousands of features in a brain scan to lead to an optimal prediction," says Dr. Kalmady.
"Machine learning and future AI (Artificial Intelligence) are approaches that enable a multi-dimensional, data-driven inroad that captures the level of complexity and objectivity that we need to unravel the wicked problems of understanding mental illness."