Data-Driven Approaches to Frailty Operationalization and Prediction of Adverse Outcomes
23 March 2022
Title:
Data-Driven Approaches to Frailty Operationalization and Prediction of Adverse Outcomes
Supervisor:
Roger A Dixon
Public
Join Zoom Meeting 9-10 am March 23
https://us02web.zoom.us/j/84871232801?pwd=VW9ISElkWWt3TkJvSDN1MUMyNzlQQT09
Meeting ID: 848 7123 2801
Passcode: 059577
Frailty is a general term referring to the accumulation with aging of multiple morbidities from such diverse domains as physiological, sensory, metabolic, mobility, and biomedical. We advance the research literature on frailty measurement and conceptualization by applying data-driven analytic technologies to multiple aging morbidity indicators. The objective was to detect clusters of deficits or individual features that elevate risk for (a) frailty emergence and progression in normal aging, (b) adverse cognitive outcomes or trajectories, and (c) applications across the Alzheimer's disease spectrum. In Study 1, we used Victoria Longitudinal Study data to examine whether baseline frailty profiles could be empirically determined and whether these extracted profiles would predict frailty progression and neurocognitive slowing. The results generalized across sex. In Study 2, we used National Alzheimer's Coordinating Center data to extract longitudinal frailty profiles (or statuses) and then characterize patterns of frailty progression in Alzheimer's disease. We next identified predictors of baseline frailty classifications and transitions. In Study 3, we used Canadian Consortium on Neurodegeneration in Aging data to identify frailty-related features that increased risk for cognitive impairment and dementia. We used machine learning prediction models to determine the differential multi-morbidity predictors of three clinical cohorts (Subjective Cognitive Impairment, Mild Cognitive Impairment, Alzheimer's disease). Understanding the impact of accumulating morbidities on adverse aging outcomes (including dementia) is a pressing research and clinical priority.
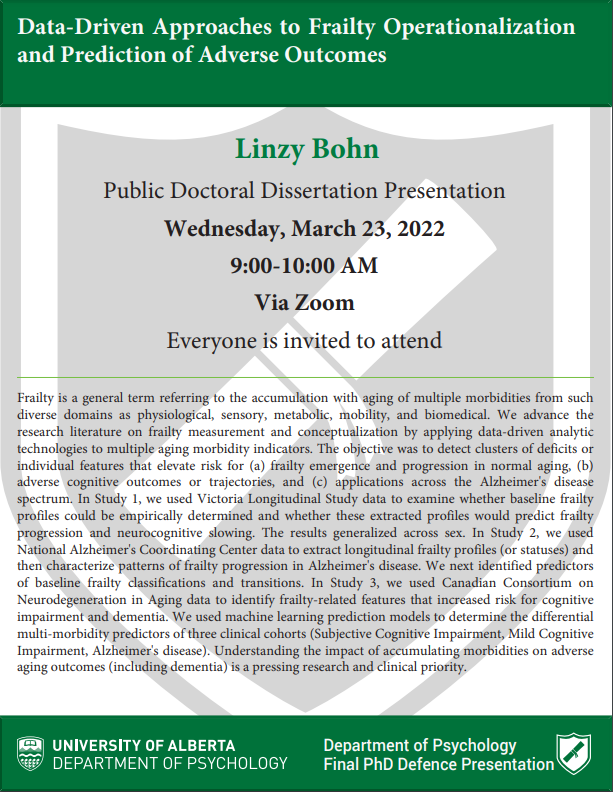