A human interest story
Oumar Salifou - 27 March 2023
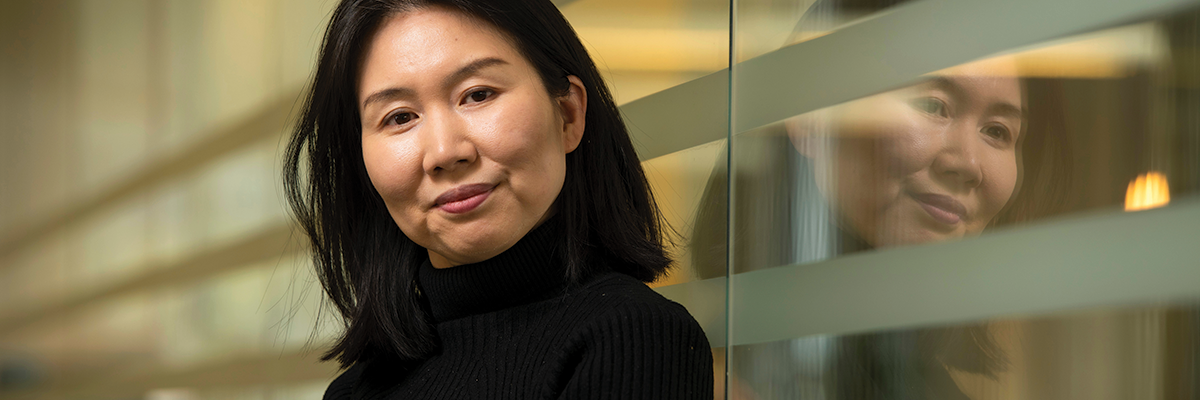
Artificial intelligence breakthroughs are, at their essence, stories about people.
Here’s one. In June 2022, Blake Lemoine was at work with Google’s LaMDA system, an AI conversation program that can keep up with human dialogue quirks and tangents. After the bot made a Star Wars joke, Lemoine was convinced LaMDA was sentient. Other human AI scientists denounced his claim and global headlines flipped from fascination with the notion of a conscious machine to Lemoine losing his job for breaching company data.
Here’s another. In February 2022, the House of Commons’ Committee on Citizenship and Immigration heard from University of Calgary law and AI professor Gideon Christian on his innovative research into the high refusal rate for foreign students from Africa applying for a Canadian study visa. He wanted to know why the Nigeria Student Express program was turning down so many students.
These are the kind of scenarios that researchers like Mi-Young Kim are chipping away at.
Kim researches the junctures of human and artificial intelligence, and the hidden ways each influences the other.
She has been involved in the field of AI since she was an undergrad in South Korea in the early 1990s. “Back then, in computing science, there was a wireless network boom,” she says. Everyone seemed to want to work on networks. “But I was more interested in the human-computer interface, and that meant AI and human language processing.” Kim is an assistant professor of computing science and a science researcher at Augustana Campus who understands the importance of focusing on humans while developing AI.
The push to improve human systems with AI and machine learning is at the crux of Kim’s labour as a scientist. She aims to make usable tools for front-line professionals and everyday people.
Kim was recruited from South Korea’s Pohang University of Science and Technology by Randy Goebel at the U of A to work as a research scientist at North Campus. With a string of collaborative projects under the Explainable Artificial Intelligence Lab (xAI Lab), at the Alberta Machine Intelligence Institute (Amii) her work is defining what it means to build computer programs that can explain their behaviours to humans, and sometimes to other systems, while creating tools that are scalable.
Goebel describes the work Kim’s team undertakes as building programs that go beyond popular computer performance programs. Computing scientists at the University of Alberta have a storied history of dominance in the games of chess, poker, checkers and Go. And as a computing professor for 40 years, when it comes to AI Goebel has seen it all.
“Studying AI was considered to be a little unusual, eccentric, sometimes even wacky,” says Goebel. “I’ve seen attitudes shift from ‘it’s a wacky discipline, why are you studying it?’ to ‘you should appreciate that you have the licence to study something that’s so eccentric,’ to ‘how could you have so carefully planned your career to be an expert at a time when AI is so important?’ ”
AI runs deep in Edmonton, which, until recently, was home to DeepMind’s first international office. DeepMind’s scientists built AlphaGo, a performance board game program that perfected the game of Go. It’s the world’s oldest continuously played board game, considered by some to be more difficult than chess to code into computers because of the way pieces flow in a fluid nature during gameplay. AlphaGo has proven that AI technology can be harvested in powerful ways to beat any human player, but the program’s code can’t explain why it made this move or that one.
“It’s not a teacher, it’s a performer,” Goebel explains. “So the foundation of the Explainable Artificial Intelligence Lab is a question. How do you capture the extra knowledge necessary in a performance computer program to allow it to entertain questions about why it makes one move rather than another? How did it think about that position?”
AI can be romantic in its unrealized promise, touted as the step past human intelligence. AI could, in theory, complete itself with a program that achieves “real” intelligence or sentience. Culturally, we have anticipated and dreaded the next leap in AI and machine learning and where it will lead humanity. Despite this, Goebel has little patience for speculation in pop culture about what AI can promise.
“When will we have conscious computers? Since you can’t define what consciousness means, I can’t answer the question for you,” he says.
Researchers like Goebel and Kim prefer to focus on the utility of AI, and they aim to build it in such a way that it can reveal its own inner decision-making processes. “If we built systems with explainability in mind we would at least be able to debug them.”
“So that’s one of the premises of our explainable AI lab,” Goebel says. “Don’t build AI systems without building them in a framework in which they represent their own information, their own knowledge, and so they can interact with humans or regulatory agencies — or whoever you like — to explain their behaviour.”
Artificial intelligence is the application of computing power to solve problems that seem to need cognitive function, like database searches, machine learning and the creation of expert systems. Artificial neural networks can be programmed, databases deepened, robots activated — and still algorithms can only go so far.
“Many people are talking about deep learning, but the next step will be collaboration with experts to surpass the limits of technology and learned data,” says Kim. “Beyond machine learning, we need humans with real expert knowledge to achieve more than the deep learning techniques can.”
Her work is funded by NSERC, Alberta Innovates and (with researcher Irene Cheng) Mitacs, and she’s seeking more funding to involve more students in her research. Currently, three graduate students are working on health projects, co-supervised with professors on North Campus.
Kim and her colleagues are working on a health project in collaboration with Alberta Health Services, and a legal project in collaboration with a new startup, Jurisage, which is a joint venture launched by Compass Law and AltaML. Both projects are developing AI that aids humans in decision-making by saving time and research effort, making hard-to-reach expertise more accessible to ordinary people. At the same time, AI would reveal the places where implicit bias exists in the data. Kim’s research areas can inform and influence how programs are built.
The legal AI she and the rest of the team are working on started when Goebel connected her to a man in Japan who was trying to pass the Japanese bar admission test. For this to happen, the machine had to understand bar exam questions, retrieve relevant statutes and compare the meaning between the question and the statute content to find an answer. “It’s one of our very complicated projects,” she says. The team developed an AI that could pass the yes/no portion of the test, based on 13 years worth of test data. Now they’re working on making the AI capable of explaining how it arrived at its answers. In the yearly Competition on Legal Information Extraction and Entailment, the team won six consecutive first-place victories in the legal bar exam test question and answer (yes/no portion) from 2014 to 2019.
Apart from Kim and Goebel, other members of the team at work on this project include Juliano Rabelo from North Campus (CIO at Jurisage) and Ken Satoh (National Institute of Informatics), Yoshinobu Kano (Shizuoka University)and Masaharu Yoshioka (Hokkaido University) in Japan. The team is connected to that startup, Jurisage, and with Compass Law and AltaML, the group is leaning into its research to develop an AI tool called MyJr, which is like an interactive legal assistant that can research case law and precedent.
“The goal is to help novice users as well as legal professionals,” Kim explains. Imagine, for example, a tenant were to sue a landlord over a breach of contract. The process might take too long and be of no financial reward once the tenant had paid a lawyer’s hourly rate. But imagine if the tenant had access to a natural language tool that let them quickly search precedent to find the likelihood of a favourable ruling? And imagine that the busy lawyer had access to a similar tool that sped up the research process, allowing them to complete the research and case work in less time, letting the AI do work that once took long hours and extra staff. Suddenly the legal system is working harder for the little guy.
And there are more serious bottlenecks in the legal system than landlord-and-tenant squabbles. Bail hearings, trials and appeals in criminal cases are essential, but there is a cost to a system so backlogged by bureaucracy and practices built in bygone times that even basic processes have bitter ends for most involved.
The price of justice in Canada takes shape in numbers.
For example, more than half of prisoners sitting in cells are without a guilty or innocent verdict — they’re just waiting for a trial in a system with delays so bad the Supreme Court has set precedents to create hard deadlines for trial dates.
To help lawyers or clients navigate thousands of pages of legal information, the AI needs to learn how to understand and apply legal precedent to give relevant predictions and advice. The machine references case law precedent with natural language processing tools, a subfield in AI that relates to how computers process large amounts of specialized human text. As the machine learns how to pull from precedent data to answer questions, its algorithm becomes stronger and it walks closer to the goal of a fully questionable platform that can help judges, lawyers and clients with advice.
For Kim, that’s the key to AI: the human in the process. When she and the team started work on the legal AI project, the aim was to help general users who cannot access lawyers because of financial barriers. A tool that takes away some of the expensive drudge work frees up legal experts to focus on work only they can do. “We wanted to create a free tool so that any person who needs legal advice can type in their concern about law, then our machines can provide relevant questions and they can also suggest some possible decisions.”
Like many health systems, Alberta’s is beyond capacity and the pressure on health-care professionals has been at a maximum since before the pandemic. Painful wait times, lack of bed space, and lack of staff due to burnout are just some of the problems hospitals contend with.
Any AI relies on data — lots of data — to learn. Lucky for Kim, Alberta Health Services archives the calls people make to 811. Kim and the team recognized that those logs represented a gold mine of data. So AHS anonymized the logs and provided them to her team so they could train the AI. The hope is that the “machine can say you need to call 911, see a doctor right now, or that you can stay at home,” says Kim.
Any AI relies on data — lots of data — to learn, and Alberta Health Services archives calls to 811. Kim and the team recognized that the logs represented a gold mine of data. And AHS anonymized the logs and provided them to her team so she could train her AI. The hope is that the “machine can say you need to call 911, see a doctor right now, or that you can stay at home,” says Kim.
The program is a complex combination of different abilities to read all anonymous patient dialogue, filter irrelevant conversational moments and jargon and then connect complaints to relevant health advice. The program could produce information that allows health services to provide feedback and training to its human staff that allows it to get more accurate health advice. The team’s program can also help professionals analyze their own patient responses to provide fair assessments for similar concerns and also notice when patients complain about certain new symptoms so that health protocols can change.
“Our team is working on the explainable AI,” she says. “We want to get the explanation rationale about the prediction, in order to help end users understand a machine’s prediction, and to help software developers debug the machine.”
Health and justice system advancements might hinge on how well they can integrate AI to create tools with built-in explainability and intelligence. For Goebel, the focus on these two areas is key and the potential to make a difference is real.
“Law and health are two areas that can actually make a difference on the planet, if you can build systems that help produce better decisions faster,” Goebel says. And Kim and her team work to create the systems in which onerous, everyday research can produce complex decisions by AI that can explain how it got there, revealing correctable biases in the process.
And in case you are wondering if the computers are coming for our jobs, the answer is yes, some jobs will be replaced by computers, but new jobs will also be created because of computing technology. Health and law are critical fields that are related to human lives, rights and well-being, so humans should intervene in the decision process. AI predictions and explanations will help professionals’ decisions and further action.
“Humans will still be in charge,” Kim says. “Ultimately, this research will improve human-AI interaction and help to build trust between users and AI.”
And for Kim, the very human element of interdisciplinary collaboration is the magic in the machine and it’s part of what attracted her to Augustana Campus. She says it helps people achieve better results from AI. “At Augustana, my colleagues are all from different educational backgrounds. When we discuss issues I can see very different views, they have different perspectives,” she says. “Sometimes, if I’m stuck in my research, I read a book about humanities,” Kim says.
“A book about humanities may have nothing to do with science, but I think all of these science studies and research are eventually about humans. By using computing science I want to help humans.”