A Convex Reformulation
A Convex Reformulation and an Outer Approximation for a Large Class of Binary Quadratic Programs
Abstract:
In this paper, we propose a general modeling and solving framework for a large class of binary quadratic programs subject to variable partitioning constraints. Problems in this class have a wide range of applications as many binary quadratic programs with linear constraints can be represented in this form. By exploiting the structure of the partitioning constraints, we propose mixed-integer nonlinear programming (MINLP) and mixed-integer linear programming (MILP) reformulations and show the relationship between the two models in terms of the relaxation strength. Our solution methodology relies on a convex reformulation of the proposed MINLP and a branch-and-cut algorithm based on outer approximation cuts, in which the cuts are generated on the fly by efficiently solving separation subproblems. To evaluate the robustness and efficiency of our solution method, we perform extensive computational experiments on various quadratic combinatorial optimization problems. The results show that our approach outperforms the state-of-the-art solver applied to different MILP reformulations of the corresponding problems.
The article, A Convex Reformulation and an Outer Approximation for a Large Class of Binary Quadratic Programs, co-authored by Borzou Rostami is a forth coming publication in Operations Research.
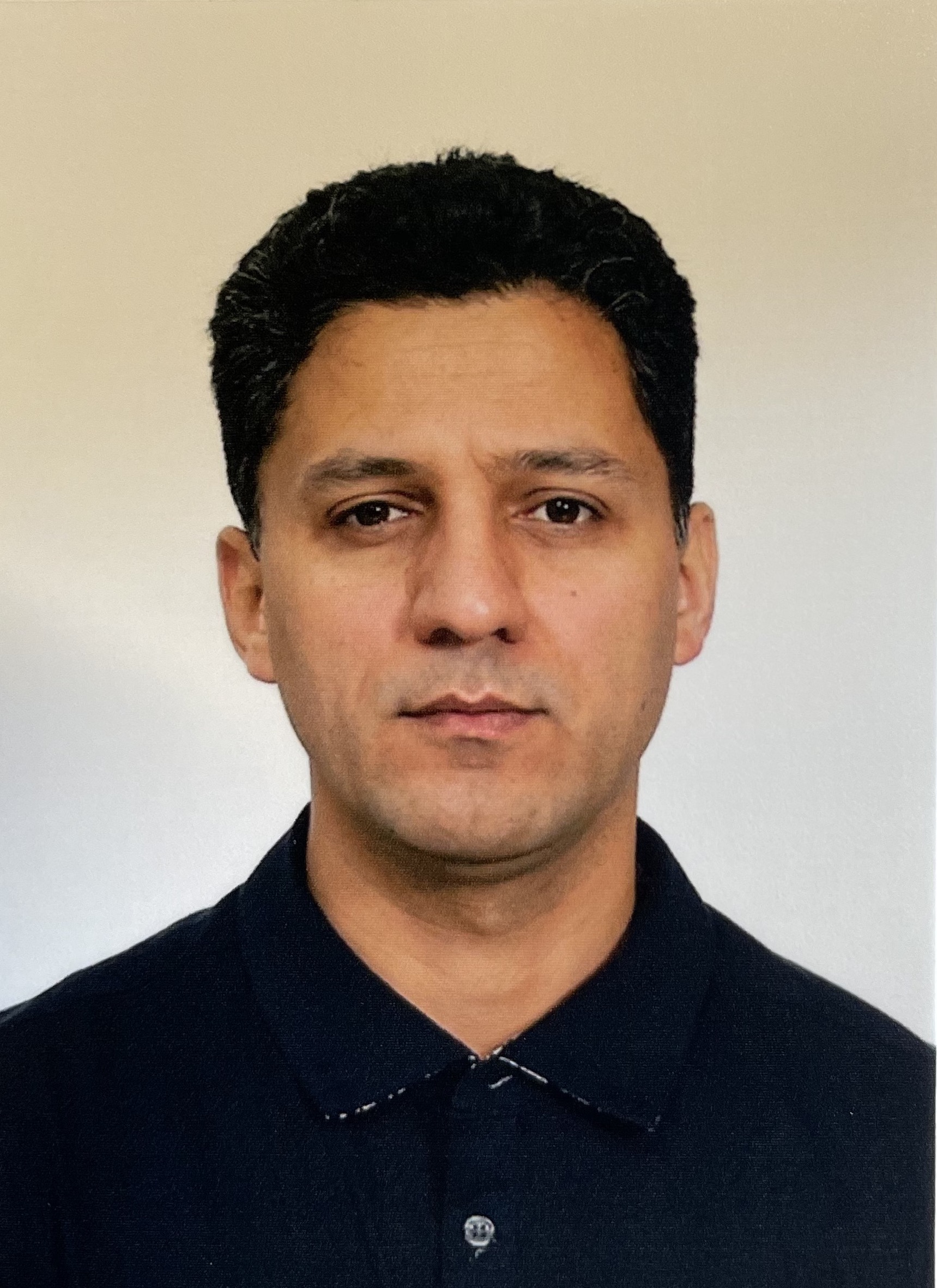
Borzou Rostami is an assistant professor and the CPA chair of Business Analytics at the Alberta School of Business, University of Alberta. He is also a member of the Canada Excellence Research Chair in Data Science for Real-Time Decision-Making (CERC DS4DM) at Polytechnique Montreal and Interuniversity Research Centre on Enterprise Networks, Logistics and Transportation (CIRRELT). Borzou holds a PhD in Information Technology/Operations Research from the Polytechnic University of Milan, Italy. Before joining the U of A, Borzou was an assistant professor at Wilfrid Laurier University and a postdoctoral researcher at Polytechnique Montreal and TU Dortmund, Germany. Borzou is currently an Associate Editor for INFOR (Information Systems and Operational Research). His research interests focus on developing optimization methods (integrated with machine learning algorithms) for large-scale decision making under data uncertainty that arise in supply chain, retail, health care, and transportation and logistics.